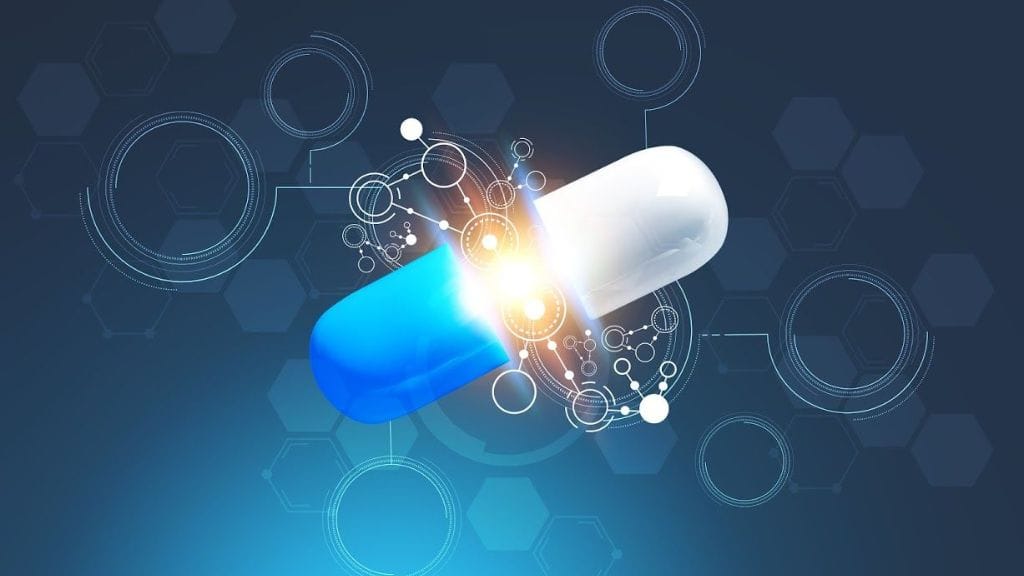
Quantum computing is rapidly transforming fields that require massive computational power, and drug discovery is no exception. With the rise of quantum machine learning (QML), pharmaceutical companies and researchers are beginning to explore a new frontier where the time and cost required to develop life-saving drugs could be drastically reduced. In this article, we’ll explore how QML is reshaping the landscape of drug discovery and its potential to solve complex biological challenges that traditional methods struggle to address.
Why Drug Discovery Needs Quantum Computing
The traditional drug discovery process is notoriously long and expensive, often taking over a decade and billions of dollars to bring a single drug to market. This is largely because researchers need to sift through vast amounts of molecular data to identify potential drug candidates. Classical computers, even with advanced machine learning algorithms, struggle to handle the complexity and scale of these problems.
Quantum computers, however, operate on the principles of quantum mechanics, enabling them to process and analyze large datasets far more efficiently than classical computers. When combined with machine learning, quantum computers can enhance our ability to model molecular interactions and predict how potential drugs will interact with the human body, a key aspect of early-stage drug discovery.
The Role of Quantum Machine Learning in Drug Discovery
Quantum machine learning integrates the power of quantum computing with the predictive capabilities of machine learning algorithms. In drug discovery, QML can be used to:
- Predict Molecular Interactions:
One of the key challenges in drug discovery is predicting how molecules interact with biological targets. QML can simulate molecular interactions with unprecedented accuracy, helping researchers identify promising drug candidates faster. - Optimize Molecular Structures:
QML can help optimize the structure of drug molecules, ensuring they bind more effectively to their targets. This optimization can drastically reduce the time it takes to identify the most promising drug compounds. - Faster Drug Screening:
Classical computers are limited in their ability to screen vast molecular libraries efficiently. QML, with its enhanced computational power, can screen these libraries much faster, identifying potential drugs that may have been overlooked by traditional methods. - Personalized Medicine:
Another exciting potential of QML in drug discovery is its role in personalized medicine. By analyzing an individual’s genetic makeup, QML can help predict how they will respond to different treatments, leading to more tailored and effective therapies.
Real-World Applications of Quantum Machine Learning in Drug Discovery
Several major pharmaceutical companies and startups are already investing in quantum computing to accelerate drug discovery. For example:
- Protein Folding: Understanding how proteins fold is critical for developing new drugs. QML models are being used to simulate protein folding more accurately, potentially unlocking new treatments for diseases such as Alzheimer’s and cancer.
- Designing New Compounds: QML can help design completely new molecular compounds that have never existed before, speeding up the development of innovative drugs.
- Virtual Drug Testing: QML is enabling the development of virtual drug testing environments, where researchers can simulate the effects of a drug on various biological systems, reducing the need for early-stage clinical trials.
Challenges and Future of QML in Drug Discovery
Despite its enormous potential, QML is still in its infancy, and several challenges remain. Quantum computers are still in the early stages of development, and creating stable, error-free quantum systems is a major hurdle. Additionally, developing QML algorithms that can run efficiently on quantum hardware is another area of active research.
However, the progress made in recent years is promising. With major tech companies such as IBM, Google, and Rigetti Computing making significant strides in quantum computing, it’s likely that we will see practical applications of QML in drug discovery within the next decade.
Conclusion
Quantum machine learning holds the potential to revolutionize the drug discovery process, cutting down both time and cost while increasing the accuracy of predictions and outcomes. As quantum computing technology advances, we can expect to see it become an essential tool in the pharmaceutical industry, unlocking new possibilities for treating complex diseases.
For those interested in the cutting edge of both quantum computing and biotechnology, the field of quantum machine learning in drug discovery is one to watch closely.
By exploring the intersection of these technologies, we are paving the way for a future where drug development becomes faster, cheaper, and more precise—ultimately leading to better health outcomes for patients around the world.