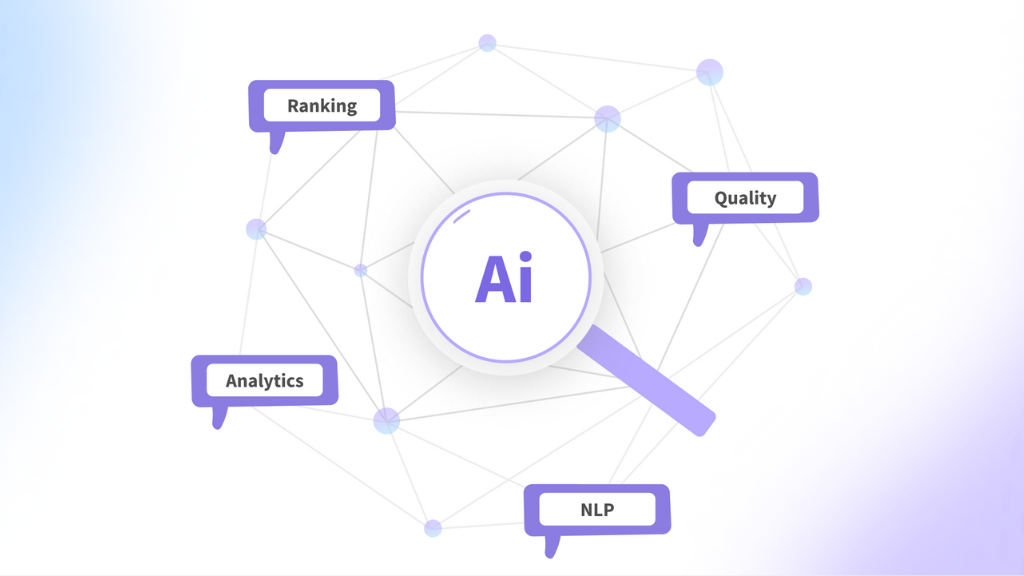
In an age dominated by information, search engines have become our go-to resources for finding answers, products, and solutions. But as information volumes increase, search engines must evolve to deliver highly relevant, accurate results. This is where artificial intelligence (AI) comes in. AI has transformed search engines into intelligent tools, helping us find what we need faster and more precisely. In this article, we’ll explore how AI-integrated search engines work, the technology behind them, and why they are so effective.
1. Understanding AI-Integrated Search Engines
An AI-integrated search engine leverages artificial intelligence technologies such as machine learning, natural language processing (NLP), and neural networks to improve search results. Unlike traditional search engines, which rely primarily on keyword matching and backlinks, AI-powered engines understand search queries on a deeper level, enabling a more refined search experience. By analyzing context, intent, and user behavior, these advanced systems can deliver results that are not only relevant but also personalized.
2. Key Technologies Behind AI in Search Engines
AI-integrated search engines rely on several core technologies to interpret and deliver optimized results:
a. Natural Language Processing (NLP)
NLP allows search engines to understand human language as we use it. With NLP, the search engine can interpret the nuances in a query, including slang, synonyms, and conversational phrases. For example, NLP helps an engine understand that “How do I fix my laptop?” is similar to “Laptop repair tips” and display similar search results.
b. Machine Learning (ML)
Machine learning models in search engines improve their results based on historical data and user interactions. ML algorithms analyze user behavior, such as click-through rates, dwell time (how long a user stays on a page), and bounce rates, to understand which results are most valuable. Over time, this data allows search engines to refine their algorithms to enhance accuracy and relevance.
c. Deep Learning and Neural Networks
Deep learning uses neural networks to process complex patterns in data. In search engines, neural networks can handle vast amounts of information and uncover intricate relationships between search terms and relevant content. This technology enables features like voice search and image recognition, which require advanced AI capabilities to match user queries accurately with the best results.
d. Knowledge Graphs
Knowledge graphs are databases of interconnected facts and entities, like people, places, and things, which help search engines generate rich snippets and answer boxes directly within search results. For example, if you search for “Albert Einstein,” the engine might show you a summary of his biography, significant achievements, and notable quotes right on the search page, thanks to the knowledge graph.
3. How AI Improves Search Intent and Context Understanding
One of the biggest challenges for traditional search engines was understanding user intent. AI-powered search engines use techniques like semantic search to determine the intent behind a query. Rather than focusing solely on individual keywords, they consider the overall meaning and context. This allows the search engine to differentiate between a product-based query (like “buy iPhone 14”) and an informational query (“how does the iPhone 14 camera work”).
AI also improves the understanding of context by analyzing previous searches, location, and even seasonality. For example, if someone searches for “best laptop” in December, the engine may prioritize holiday deals in the results. Similarly, a person searching for “pizza places” while walking downtown will see results for nearby pizzerias.
4. Personalization Through AI
AI-integrated search engines can personalize search results by analyzing user behavior and preferences. Factors like past searches, browsing history, and demographics play a significant role in tailoring the experience. Google, for instance, uses machine learning models like RankBrain and BERT to offer results based on individual user patterns and needs. This personalization ensures that each user’s search experience feels unique and relevant to their current interests.
5. Enhancing Visual and Voice Search Capabilities
Search engines have evolved beyond text. Visual search, powered by AI, allows users to search using images instead of text, which is particularly useful for identifying products or landmarks. Voice search, another innovation, uses speech recognition to interpret and process spoken queries. Technologies like Google’s Assistant and Amazon’s Alexa are prime examples of AI-driven voice search, capable of understanding spoken language nuances and context for a smooth, hands-free search experience.
6. Handling Content Quality and Misinformation
In today’s digital landscape, misinformation is a significant challenge. AI-integrated search engines are equipped with tools to identify and filter out low-quality content. By analyzing factors such as content structure, source credibility, and user engagement, these engines prioritize high-quality, credible sources. Google’s E-A-T (Expertise, Authoritativeness, Trustworthiness) guidelines are a prominent example of this effort to improve the quality of search results.
7. Continuous Improvement Through Feedback Loops
AI-powered search engines are designed to improve over time through feedback loops. When users interact with search results, their actions—such as clicks, shares, and time spent on a page—are analyzed by machine learning models. The feedback gathered is used to continuously refine algorithms, making search engines smarter and more accurate with each interaction.
8. Challenges and Future of AI-Integrated Search Engines
While AI integration has significantly improved search engines, challenges remain. Privacy concerns are paramount, as personalization often relies on tracking user behavior. There’s also the issue of algorithmic bias, where machine learning models may inadvertently favor certain types of content. To address these issues, companies are developing transparency and accountability measures in their algorithms, along with privacy-preserving techniques like federated learning, which processes data locally on the device instead of centrally.
The future of AI-integrated search engines lies in even greater contextual awareness, multimodal search (text, voice, and visual), and deeper personalization. The rise of AI models like OpenAI’s GPT and LLMs (large language models) promises a more conversational and intuitive search experience that understands complex, multi-part questions and interacts with users in real time.
Conclusion: The Power of AI in Search
AI-integrated search engines represent a monumental shift in how we find information online. By incorporating NLP, machine learning, neural networks, and more, these engines have transcended basic keyword matching to offer a highly personalized, context-aware, and accurate search experience. As AI technologies continue to advance, we can expect search engines to become even more attuned to our needs, providing an invaluable tool for navigating the vast expanse of information available at our fingertips.